Artificial intelligence (AI) can transform products, customer experiences, and entire business models.
But, as anyone who’s ventured into the world of AI knows firsthand, there are no guarantees. Putting AI to work requires a holistic view, from the way you incorporate data science into your organization, to the approach you take to product and experience design, to the makeup of the teams who execute your strategy.
Here are four ways you can help your organization get AI off your roadmap and into your products for the benefit of your customers – and your business.
1. Instill a common data science mission across the company
It’s commonly understood by most of us that successful AI initiatives depend on technology, people, and data, brought together in just the right way—but that doesn’t stop many companies from emphasizing one above the others. Some focus narrowly on building the best possible technology; others assemble a world-class team and trust them to deliver; still others aim to gather the most data possible and hope the rest will fall into place.
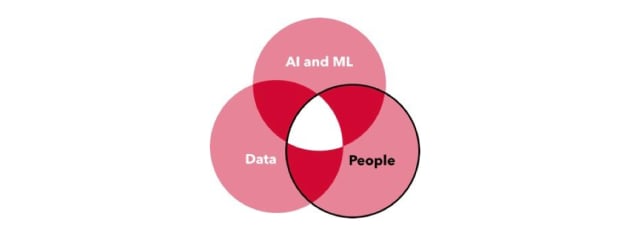
There’s one thing I know for sure: the magic happens when all three components are in balance.
One way to ensure that technology, people, and data come together in the right way is to build data science into the very fabric of your company. Clear and consistent communication about the mission of the organization, celebration of AI implementation successes – and customer benefits – are critical to achieving a high-functioning team of data scientists, data analysts and platform partners. Regardless of how you structure your organization, instilling a common purpose, mission, values, and culture is key to keeping everyone on the same page without being overly focused on or influenced by individual business units or functional groups.
2. Incorporate design thinking
Intuit’s Design for Delight philosophy is grounded in deep customer empathy. Technology should never drive product design. It’s essential to begin by cultivating an understanding of the customer’s needs, and only then apply technology in service of those needs. By seeing the world through the eyes of your customers, and understanding their problems from their own perspective, you can provide products and experiences that make the right kind of difference for them. For example, let’s say you’re a baker in Des Moines, Iowa. What are you trying to do? The answer is not, “I’m trying to use a deep learning algorithm to solve baking problems.” The answer is more along the lines of, “I want to sell more cupcakes.” If you can’t write a problem statement that direct and relevant, there’s a good chance you’re building a solution to the wrong problem—or no problem at all—and just applying technology for its own sake.
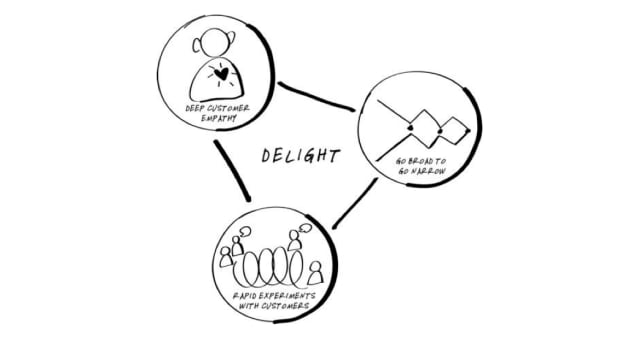
We also focus on going broad, then going narrow. Our teams come up with a large number of ideas to explore, and then ask which ones can work from an AI perspective. At that point, we can move to rapid prototyping and A/B testing. This is where machine learning at scale gets exciting, as we gather learnings from live customer interactions to further refine and enhance our AI projects. An AI innovation challenge can generate even more ideas to work with. See what kind of AI and machine learning ideas your whole staff can come up with—but make sure each is accompanied by the kind of concrete problem statement our Des Moines baker had.
3. Infuse a product management mindset into your data scientists (and vice versa)
It can be easy for product managers and data scientists to develop an “us-and-them” mentality. Data scientists might feel that their educational background gives them a privileged understanding of the right way to proceed. On the other hand, product managers might conclude that their years of real-world experience with products and customers should carry more weight than any data-derived abstraction or algorithm. This standoff is highly unproductive; product management and data science must work together to bring the right products to market.
At Intuit, we bring together people from four interrelated disciplines to make collective decisions about what goes into the product: product development, experience design, product management, and data science. We also offer data science training opportunities to create a common language for day-to-day collaboration across organizational boundaries and disciplines.
4. Build a diverse, inclusive team
Diversity and inclusion are obviously important as human values, but they also make good business sense. It’s important for technologists to realize that there’s a big world out there that we can’t know every part of, and that we need to have a broad mix of people on our teams to help fill in those gaps in knowledge and understanding. Diversity applies across many dimensions—gender, generation, personal background, profession, point of view, and so on. The more kinds of people you have on your team, the more ways they’ll be able to come up with to solve problems and reach goals. That’s especially important given that the solution that works for one kind of person might not be as effective for another. If you only have that one kind of person, you’ll never see your blind spots — until you get to market, only to discover that you’ve left some of your customers with unsolved problems. If you don’t represent someone’s point of view, it’s going to be hard to build the right AI system for them.
Once you have these elements in place in your organization, your AI capability can really take off, as you originate, iterate, and test ideas, and tune the algorithms and data flows that operationalize them in your products.
You’ll notice that I haven’t said much about deep learning, natural language processing, natural language understanding, decision making under uncertainty, automated reasoning, or other critical components of a production-ready AI system. Technologies like these are of course important, but it’s a trap to deep-dive into the systems level without first thinking about the big picture: what it takes from a holistic standpoint to build products that solve real customer problems the right way. That’s the key to sustainable, durable success in the world of AI.